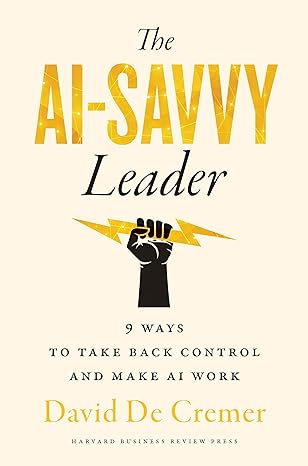
The AI-Savvy Leader: Nine Ways to Take Back Control and Make AI Work - By David De Cremer
Date read: 2024-07-03How strongly I recommend it: 5/10
(See my list of 150+ books, for more.)
Go to the Amazon page for details and reviews.
Meant to help leaders understand how to implement AI, it mostly focused on basic change management skills that leaders should use with any major transformation initiative. There are many other books out there that explain these concepts better and not enough AI content to recommend.
My Notes
Investments in digital transformation were projected to top $6.8 trillion by 2023, but a staggering 87 percent of these projects thus far are failing to meet their intended objectives.
At a basic level, AI systems are computational systems that have a self-learning ability. In other words, AI can learn from large datasets and engage in pattern recognition and problem-solving. AI thus has the ability to analyze large and complex datasets to reveal underlying trends, on which it can base its recommendations.
In your continuous learning trajectory, you will need to stay up to date on the responsibilities that come along with AI adoption.
Business leaders need to oversee the available data and know its quality, manage the insights inferred from it, and ensure that the insights answer the right questions.
Including employees in the design and deployment of AI systems, for instance, can dramatically improve their feelings of control. By incorporating employees’ perspectives and feedback, you can design AI systems in ways that are intuitive, user-friendly, and supportive of people’s daily tasks and responsibilities.
Tear down those silos and build diverse teams that work together to adopt AI. For example, business experts can explain to tech experts what business goals need to be achieved, and tech experts can make suggestions regarding which AI systems will have to be used.
Leaders need to ensure quicker information flow by creating the opposite of the preceding communication structure: make the organization flat so that more people are part of the conversation and there’s more bottom-up information flow and fewer administrative bottlenecks from middle layers of management.
Listen carefully to the experts: those who know the tech inside and out and who can help you explain it to nonexperts in a way that is both accurate and approachable.
Questions to ask Data Scientists:
- What, in clear and simple terms, are the technical features of the AI system we are looking to adopt?
- What technical measures can be taken to keep the system functioning robustly and safely?
- What are some future trends in AI development that I should be keeping a lookout for?
- What are some pressing issues in your domain that might benefit from an AI-based solution?
- What existing best practices might be disrupted by an AI solution, and what can we do to preserve them?
As an AI-savvy leader, you should seek to set in place policies and internal governance procedures to remain ahead of the regulatory curve. By incorporating ethical and social implications into your strategic vision, you can navigate your AI adoption journey with integrity, foresight, and responsibility.
One useful approach is to work like Hugging Face, a French American company and open-source community. The company’s large, dedicated, and well-resourced AI ethics group is composed of a diverse assemblage of technical, legal, philosophical, and business experts with a broad mandate to intervene effectively across a wide range of the company’s AI service offerings.17 By design, this group is dispersed across the organization, with the belief that it is important to have a shared responsibility and commitment from all parties involved to acknowledge and learn about the ethical stakes of the company’s work. In extreme cases, the ethics group can come together to call for projects to be stopped if the ethical impact assessment reveals significant material harm. In most other cases, the group helps projects across the company think through and deal with potential risks.
A human-centered approach to adopting AI: But if your AI adoption process is motivated largely by the maximization of profits and efficiency, you will then create a culture where humans feel pressured to align with how machines make decisions. Such an approach lacks any respect for the human condition and will ultimately work against you. With this strategy, people work around the technology, results don’t materialize, turnover increases, innovation declines, and your reputation in the market suffers to the extent that good human talent wants nothing to do with your company.
Modifying AI to account for human behavior, Alibaba packing example: The researchers, however, included an experimental condition in which they adjusted the algorithm by accounting for the workers’ tendency to use larger bins and their other preferences. When this human-centered bin-packing algorithm was put to work, the results were astonishing. Simply by anticipating and incorporating human behavior, the human-centered AI made the workers deviate less from the optimization model, and it successfully improved their performance (i.e., the average packing time of targeted packages decreased).
Automation efforts take over the jobs that humans waste their time on. With these tasks automated, people can grow by working in more creative ways and can hence contribute to innovation and productivity. The focus of any AI adoption project, therefore, is to develop employees by upskilling them in the areas that define them as human beings.
What you want most is for your employees to get better at one key ability that humans develop early on in life and that companies benefit the most from: creativity. Creativity requires a job situation where employees are encouraged to imagine, critically evaluate new ideas, and reflect on them.
As many change consultants will tell you, when organizations start their AI adoption project, they usually spend up to 90 percent of their budget on the technology itself. The consequence is that little money is left to put AI to work in collaboration with the workforce.
According to Deloitte, soft-skill-intensive occupations will grow at 2.5 times the rate of jobs in other fields and will account for two-thirds of all jobs by 2030.
Constantly ask questions to see if the right data is available to provide useful answers and accurate predictions. If not, then you need to grow your datasets. The questions you keep asking—as a leader with good hard and soft skills—constitute an important catalyst in evaluating data and pushing the tech experts to help find solutions to new questions.