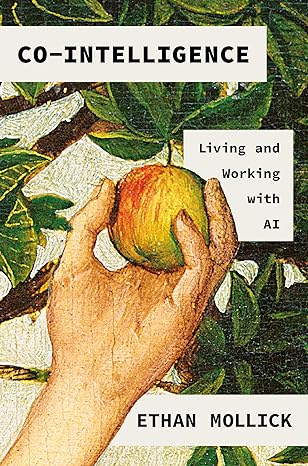
Co-Intelligence: Living and Working with AI - By Ethan Mollick
Date read: 2024-09-02How strongly I recommend it: 8/10
(See my list of 150+ books, for more.)
Go to the Amazon page for details and reviews.
Good book for those trying to understand how to apply AI to your life. Covers best practices when using AI (mostly LLMs) and includes predictions of how AI will evolve over time.
Contents:
My Notes
You should try inviting AI to help you in everything you do, barring legal or ethical barriers. As you experiment, you may find that AI help can be satisfying, or frustrating, or useless, or unnerving. But you aren’t just doing this for help alone; familiarizing yourself with AI’s capabilities allows you to better understand how it can assist you—or threaten you and your job.
A fundamental truth about innovation: it is expensive for organizations and companies but cheap for individuals doing their job.
Innovation comes from trial and error, which means that an organization trying to launch a new product to help a marketer write more compelling copy would need to build the product, test it on many users, and make changes many times to make something that works. A marketer, however, is writing copy all the time and can experiment with many different ways of using AI to help until they find one that succeeds. No need to hire a team or use expensive software development cycles.
Working with AI is easiest if you think of it like an alien person rather than a human-built machine.
The key is to give the LLM some guidance and direction on how to generate outputs that match your expectations and needs, to put it in the right “headspace” to give you interesting and unique answers. For example, you could also ask it to act as an expert, a friend, a critic, a storyteller, or any other role that suits your purpose.
So, by embracing this principle, you can view AI’s limitations as transient, and remaining open to new developments will help you adapt to change, embrace new technologies, and remain competitive in a fast-paced business landscape driven by exponential advances in AI.
Treating AI as a person, then, is more than a convenience; it seems like an inevitability, even if AI never truly reaches sentience. We seem to be willing to fool ourselves into seeing consciousness everywhere, and AI will certainly be happy to help us do so.
Why LLMs Hallucinate: Different LLMs may have different architectures, training data, and objectives. But in many ways, hallucinations are a deep part of how LLMs work. They don’t store text directly; rather, they store patterns about which tokens are more likely to follow others. That means the AI doesn’t actually “know” anything. It makes up its answers on the fly. Plus, if it sticks too closely to the patterns in its training data, the model is said to be overfitted to that training data. Overfitted LLMs may fail to generalize to new or unseen inputs and generate irrelevant or inconsistent text—in short, their results are always similar and uninspired. To avoid this, most AIs add extra randomness in their answers, which correspondingly raises the likelihood of hallucination.
As creative as the AI can be, without careful prompting, the AI tends to pick similar ideas every time. The concepts may be good, even excellent, but they can start to seem a little same-y after seeing enough of them. Thus, a large group of creative humans will usually generate a wider diversity of ideas than the AI. All of this suggests that humans still have a large role to play in innovation . . . but that they would be foolish not to include AI in that process, especially if they don’t consider themselves highly creative.
We will get even better quality if we remember the principle of telling AI who it is: You are an expert at marketing. When asked to generate slogan ideas you come up with ideas that are different from each other, clever, and interesting. You use clever wordplay. You try not to repeat themes or ideas. Come up with 20 ideas for marketing slogans for a new mail-order cheese shop, make them different from each other, and make them clever and creative.
Another prompt example to inspire creativity: You are an expert at problem-solving and idea generation. When asked to solve a problem, you come up with novel and creative ideas. Tell me 10 detailed ways a superhero might make espresso and how they might speculatively get the same effects in a new product.
It is important to stick with the principle of inviting AI to everything, letting us learn the shape of the Jagged Frontier and how it maps onto the unique complex of tasks that comprise our individual jobs. With that knowledge, we need to be conscious about the tasks we are giving AI, so as to take advantage of its strengths and our weaknesses.
We divide our tasks into categories that are more or less suitable for AI disruption.
Just Me Tasks are not going to be static; they can change as AI evolves and as preferences shift. The key is to recognize the tasks that are meaningful and fulfilling for you as a human being and that you would rather not delegate or share with an AI system.
The next category of tasks is Delegated Tasks. These are tasks that you assign the AI and may carefully check (remember, the AI makes stuff up all the time), but ultimately do not want to spend a lot of time on. This is usually stuff you really don’t want to do and is of low importance, or time-consuming. The perfect Delegated Task is tedious, repetitive, or boring for humans but easy and efficient for AI.
Then there are Automated Tasks, ones you leave completely to the AI and don’t even check on. Perhaps there is a category of email that you just let AI deal with, for example.
One approach, called chain-of-thought prompting, gives the AI an example of how you want it to reason, before you make your request. Even more usefully, you can also provide step-by-step instructions that build on each other, making it easier to check the output of each step (letting you refine the prompt later), and which will tend to make the output of your prompts more accurate.
For example, think this through step by step: come up with good analogies for an AI tutor. First, list possible analogies. Second, critique the list and add three more analogies. Next, create a table listing pluses and minuses of each. Next, pick the best and explain it.
In field after field, we are finding that a human working with an AI co-intelligence outperforms all but the best humans working without an AI.