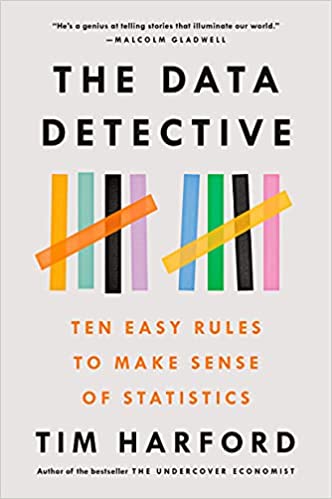
The Data Detective: Ten Easy Rules to Make Sense of Statistics - by Tim Harford
Date read: Y2022-08-27How strongly I recommend it: 6/10
(See my list of 150+ books, for more.)
Go to the Amazon page for details and reviews.
Good to help you ask the right questions before believing a study or newspaper headline discussing a recent study. I wish it would have helped me better interpret actual scientific studies, though.
Contents:
- RULE ONE - SEARCH YOUR FEELINGS
- RULE TWO - PONDER YOUR PERSONAL EXPERIENCE
- RULE THREE - AVOID PREMATURE ENUMERATION
- RULE FOUR -STEP BACK AND ENJOY THE VIEW
- RULE FIVE - GET THE BACKSTORY
- RULE SIX - ASK WHO IS MISSING
- RULE SEVEN - DEMAND TRANSPARENCY (AI/ML)
- RULE NINE - REMEMBER THAT MISINFORMATION CAN BE BEAUTIFUL, TOO
- RULE TEN - KEEP AN OPEN MIND
My Notes
When it comes to interpreting the world around us, we need to realize that our feelings can trump our expertise.
We often find ways to dismiss evidence that we don’t like. And the opposite is true, too: when evidence seems to support our preconceptions, we are less likely to look too closely for flaws.
We don’t need to become emotionless processors of numerical information—just noticing our emotions and taking them into account may often be enough to improve our judgment. Rather than requiring superhuman control over our emotions, we need simply to develop good habits. Ask yourself: How does this information make me feel? Do I feel vindicated or smug? Anxious, angry, or afraid? Am I in denial, scrambling to find a reason to dismiss the claim?
People with deeper expertise are better equipped to spot deception, but if they fall into the trap of motivated reasoning, they are able to muster more reasons to believe whatever they really wish to believe.
The counterintuitive result is that presenting people with a detailed and balanced account of both sides of the argument may actually push people away from the center rather than pull them in. If we already have strong opinions, then we’ll seize upon welcome evidence, but we’ll find opposing data or arguments irritating. This biased assimilation of new evidence means that the more we know, the more partisan we’re able to be on a fraught issue.
Psychologists have a name for our tendency to confuse our own perspective with something more universal: it’s called “naive realism,” the sense that we are seeing reality as it truly is, without filters or errors.
Social scientists have long understood that statistical metrics are at their most pernicious when they are being used to control the world, rather than try to understand it. Economists tend to cite their colleague Charles Goodhart, who wrote in 1975: “Any observed statistical regularity will tend to collapse once pressure is placed upon it for control purposes.” (Or, more pithily: “When a measure becomes a target, it ceases to be a good measure.”)
Try to take both perspectives—the worm’s-eye view as well as the bird’s-eye view. They will usually show you something different, and they will sometimes pose a puzzle: How could both views be true? That should be the beginning of an investigation. Sometimes the statistics will be misleading, sometimes it will be our own eyes that deceive us, and sometimes the apparent contradiction can be resolved once we get a handle on what is happening.
The whole discipline of statistics is built on measuring or counting things.
Michael Blastland, co-creator of More or Less, imagines looking at two sheep in a field. How many sheep in the field? Two, of course. Except that one of the sheep isn’t a sheep, it’s a lamb. And the other sheep is heavily pregnant—in fact, she’s in labor, about to give birth at any moment. How many sheep again? One? Two? Two and a half? Counting to three just got difficult.
They had dived into the mathematics of a statistical claim—asking about sampling errors and margins of error, debating if the number is rising or falling, believing, doubting, analyzing, dissecting—without taking the time to understand the first and most obvious fact: What is being measured, or counted? What definition is being used?
Our confusion often lies less in numbers than in words. Before we figure out whether nurses have had a pay raise, first find out what is meant by “nurse.” Before lamenting the prevalence of self-harm in young people, stop to consider whether you know what “self-harm” is supposed to mean.
Another way to step back and enjoy the view is to give yourself a sense of scale. Faced with a statistic, simply ask yourself, “Is that a big number?”
Andrew Elliott—an entrepreneur who likes the question so much he published a book with the title Is That a Big Number?—suggests that we should all carry a few “landmark numbers” in our heads to allow easy comparison. A few examples:
We are drawn to surprising news, and surprising news is more often bad than good. But the psychologist Steven Pinker has argued that good news tends to unfold slowly, while bad news is often more sudden.
This particular flavor of survivorship bias is called “publication bias.” Interesting findings are published; non-findings, or failures to replicate previous findings, face a higher publication hurdle.
So not only are journals predisposed to publish surprising results, researchers facing “publish or perish” incentives are more likely to submit surprising results that may not stand up to scrutiny.
If a particular way of analyzing the data produces no result, and a different way produces something more intriguing, then of course the more interesting method is likely to be what is reported and then published.
Scientists sometimes call this practice “HARKing”—HARK is an acronym for Hypothesizing After Results Known.
Ask yourself if the journalist reporting on the research has clearly explained what’s being measured. Was this a study done with humans? Or mice? Or in a petri dish? A good reporter will be clear.
How large is the effect? Was this a surprise to other researchers? A good journalist will try to make space to explain—and the article will be much more fun to read as a result, satisfying your curiosity and helping you to understand.
If in doubt, you can easily find second opinions: almost any major research finding in science or social science will quickly be picked up and digested by academics and other specialists, who’ll post their own thoughts and responses online.
How large is the effect? Was this a surprise to other researchers? A good journalist will try to make space to explain—and the article will be much more fun to read as a result, satisfying your curiosity and helping you to understand.
If the story you’re reading is about health, there’s one place you should be sure to look for a second opinion: the Cochrane Collaboration.
A related network, the Campbell Collaboration, aims to do the same thing for social policy questions in areas such as education and criminal justice.
We should draw conclusions about human nature only after studying a broad range of people. Psychologists are increasingly acknowledging the problem of experiments that study only “WEIRD” subjects—that is, Western, Educated, and from Industrialized Rich Democracies.
Consider the historical underrepresentation of women in clinical trials.
A different problem arises when women are included in data-gathering exercises but the questions they are asked don’t fit the man-shaped box in the survey designer’s head.
An even subtler gap in the data emerges from the fact that governments often measure the income not of individuals but of households.
And yet while many households pool their resources, we cannot simply assume that they all do: money can be used as a weapon within a household, and unequal earnings can empower abusive relationships. Collecting data on household income alone makes such abuse statistically invisible, irrelevant by definition. It is all too tempting to assume that what we do not measure simply does not exist.
Sample error reflects the risk that, purely by chance, a randomly chosen sample of opinions does not reflect the true views of the population. The “margin of error” reported in opinion polls reflects this risk, and the larger the sample, the smaller the margin of error. A thousand interviews is a large enough sample for many purposes, and during the 1936 election campaign Mr. Gallup is reported to have conducted three thousand interviews.
Sampling error is when a randomly chosen sample doesn’t reflect the underlying population purely by chance; sampling bias is when the sample isn’t randomly chosen at all.
The missing responses are examples of what the statistician David Hand calls “dark data”: we know the people are out there and we know that they have opinions, but we can only guess at what those opinions are. We can ignore dark data, as Asch and Milgram ignored the question of how women would respond in their experiments, or we can try desperately to shine a light on what’s missing. But we can never entirely solve the problem.
Big found datasets can seem comprehensive, and may be enormously useful, but “N = All” is often a seductive illusion: it’s easy to make unwarranted assumptions that we have everything that matters. We must always ask who and what is missing.
Onora O’Neill argues that if we want to demonstrate trustworthiness, we need the basis of our decisions to be “intelligently open.” She proposes a checklist of four properties that intelligently open decisions should have. Information should be accessible: that implies it’s not hiding deep in some secret data vault. Decisions should be understandable—capable of being explained clearly and in plain language. Information should be usable—which may mean something as simple as making data available in a standard digital format. And decisions should be assessable—meaning that anyone with the time and expertise has the detail required to rigorously test any claims or decisions if they wish to.
Ideas that are best expressed in words or numbers are turned into graphics anyway, because that’s what spreads on social media. Unfortunately, the selection mechanism is often some combination of beauty and shock value, rather than pertinence and accuracy.
First—and most important, since the visual sense can be so visceral—check your emotional response. Pause for a moment to notice how the graph makes you feel: triumphant, defensive, angry, celebratory? Take that feeling into account.
Second, check that you understand the basics behind the graph. What do the axes actually mean? Do you understand what is being measured or counted? Do you have the context to understand, or is the graph showing just a few data points? If the graph reflects complex analysis or the results of an experiment, do you understand what is being done? If you’re not in a position to evaluate that personally, do you trust those who were? (Or have you, perhaps, sought a second opinion?).
Curiosity breaks the relentless pattern. Specifically, Kahan identified “scientific curiosity.” That’s different from scientific literacy. The two qualities are correlated, of course, but there are curious people who know rather little about science (yet), and highly trained people with little appetite to learn more.
There’s a sweet spot for curiosity: if we know nothing, we ask no questions; if we know everything, we ask no questions either. Curiosity is fueled once we know enough to know that we do not know.
In a world where so many people seem to hold extreme views with strident certainty, you can deflate somebody’s overconfidence and moderate their politics simply by asking them to explain the details.