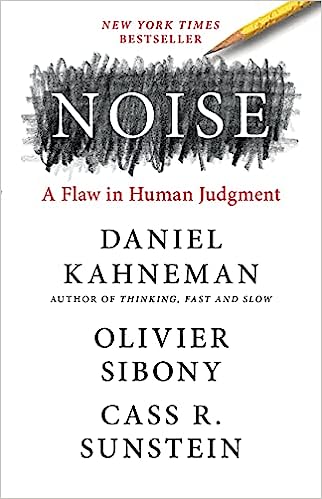
Noise: A Flaw in Human Judgment - by Daniel Kahneman, Olivier Sibony, and Cass R. Sunstein
Date read: 2021-06-05How strongly I recommend it: 6/10
(See my list of 150+ books, for more.)
Go to the Amazon page for details and reviews.
Daniel Kahneman's follow-up book from Thinking Fast & Slow; not as good as his first book and clearly different chapters were written by different authors. The majority of the book goes into granular detail to define noise (which is different than bias) and how to measure it in situations such as courtrooms, hospitals, and in business. The most actionable items are included in Part V where Kahneman provides advice on how to reduce noise when it comes to making judgements such as interviewing and deciding on acquisitions.
Contents:
- DEFINING NOISE
- HOW TO MEASURE NOISE
- NOISE IN PREDICTIVE JUDGEMENTS
- HOW NOISE HAPPENS
- IMPROVING JUDGEMENTS
- PERFORMANCE RATINGS
- INTERVIEWING
- ACQUISITION JUDGEMENT EXAMPLE
- OPTIMAL NOISE
My Notes
Bias and noise — systematic deviation and random scatter — are different components of error.
Some judgments are biased; they are systematically off target. Other judgments are noisy, as people who are expected to agree end up at very different points around the target. Many organizations, unfortunately, are afflicted by both bias and noise.
A general property of noise is that you can recognize and measure it while knowing nothing about the target or bias.
Wherever there is judgment, there is noise—and more of it than you think.
“Other people view the world much the way I do.” These beliefs, which have been called naive realism, are essential to the sense of a reality we share with other people. We rarely question these beliefs. We hold a single interpretation of the world around us at any one time, and we normally invest little effort in generating plausible alternatives to it. One interpretation is enough, and we experience it as true. We do not go through life imagining alternative ways of seeing what we see.
How had the leaders of the company remained unaware of their noise problem? There are several possible answers here, but one that seems to play a large role in many settings is simply the discomfort of disagreement. Most organizations prefer consensus and harmony over dissent and conflict.
We cannot measure noise in a singular decision, but if we think counterfactually, we know for sure that noise is there.
A singular decision is a recurrent decision that happens only once. Whether you make a decision only once or a hundred times, your goal should be to make it in a way that reduces both bias and noise. And practices that reduce error should be just as effective in your one-of-a-kind decisions as in your repeated ones.
Scholars of decision-making offer clear advice to resolve this tension: focus on the process, not on the outcome of a single case.
A reduction of noise has the same impact on overall error as does a reduction of bias by the same amount. For that reason, the measurement and reduction of noise should have the same high priority as the measurement and reduction of bias.
Gauss proposed a rule for scoring the contribution of individual errors to overall error. His measure of overall error—called mean squared error (MSE)—is the average of the squares of the individual errors of measurement. For instance, if the true value was 971, the errors in the five measurements would be 0, 1, 2, 8, and 9. The squares of these errors add up to 150, and their mean is 30. This is a large number, reflecting the fact that some measurements are far from the true value. You can see that MSE decreases as we get closer to 975—the mean—and increases again beyond that point. The mean is our best estimate because it is the value that minimizes overall error.
When your estimate increases by just 3 millimeters, from 976 to 979, for instance, MSE doubles. This is a key feature of MSE: squaring gives large errors a far greater weight than it gives small ones.
Error in a single measurement = Bias + Noisy Error
Overall Error (MSE) = Bias2 + Noise2
Bias and noise are interchangeable in the error equation, and the decrease in overall error will be the same, regardless of which of the two is reduced.
In terms of overall error, noise and bias are independent: the benefit of reducing noise is the same, regardless of the amount of bias.
System noise is undesirable variability in the judgments of the same case by multiple individuals.
Level noise is variability in the average level of judgments by different judges.
Pattern noise is variability in judges’ responses to particular cases.
Two researchers, Edward Vul and Harold Pashler, had the idea of asking people to answer this question (and many similar ones) not once but twice. The subjects were not told the first time that they would have to guess again. Vul and Pashler’s hypothesis was that the average of the two answers would be more accurate than either of the answers on its own. The data proved them right. In general, the first guess was closer to the truth than the second, but the best estimate came from averaging the two guesses.
The wisdom-of-crowds effect: averaging the independent judgments of different people generally improves accuracy.
Instead of merely asking their subjects to produce a second estimate, they encouraged people to generate an estimate that—while still plausible—was as different as possible from the first one.
The instructions to participants read as follows: First, assume that your first estimate is off the mark. Second, think about a few reasons why that could be. Which assumptions and considerations could have been wrong? Third, what do these new considerations imply? Was the first estimate rather too high or too low? Fourth, based on this new perspective, make a second, alternative estimate.
Creating space produced larger improvements in accuracy than did a simple request for a second estimate immediately following the first. Because the participants forced themselves to consider the question in a new light, they sampled another, more different version of themselves—two “members” of the “crowd within” who were further apart. As a result, their average produced a more accurate estimate of the truth.
Inducing good moods makes people more receptive to bullshit and more gullible in general; they are less apt to detect deception or identify misleading information. Conversely, eyewitnesses who are exposed to misleading information are better able to disregard it—and to avoid false testimony—when they are in a bad mood.
Gambler's Fallacy: After a streak, or a series of decisions that go in the same direction, they are more likely to decide in the opposite direction than would be strictly justified. As a result, errors (and unfairness) are inevitable. We tend to underestimate the likelihood that streaks will occur by chance.
Social influences create significant noise across groups.
While multiple independent opinions, properly aggregated, can be strikingly accurate, even a little social influence can produce a kind of herding that undermines the wisdom of crowds.
A regression model is too successful in the original sample, and a cross-validated correlation is almost always lower than it was in the original data.
You can make valid statistical predictions without prior data about the outcome that you are trying to predict. All you need is a collection of predictors that you can trust to be correlated with the outcome.
The combination of two or more correlated predictors is barely more predictive than the best of them on its own.
Simple rules that can be applied with little or no computation have produced impressively accurate predictions in some settings, compared with models that use many more predictors.
It is possible, and perhaps too easy, to build an algorithm that perpetuates racial or gender disparities, and there have been many reported cases of algorithms that did just that. The visibility of these cases explains the growing concern about bias in algorithmic decision making. Before drawing general conclusions about algorithms, however, we should remember that some algorithms are not only more accurate than human judges but also fairer.
Resistance to algorithms, or algorithm aversion, does not always manifest itself in a blanket refusal to adopt new decision support tools. More often, people are willing to give an algorithm a chance but stop trusting it as soon as they see that it makes mistakes. On one level, this reaction seems sensible: why bother with an algorithm you can’t trust? As humans, we are keenly aware that we make mistakes, but that is a privilege we are not prepared to share. We expect machines to be perfect. If this expectation is violated, we discard them.
When comparing any two candidates, what is the probability that the one you thought had more potential did in fact turn out to be the higher performer? We often informally poll groups of executives on this question. The most frequent answers are in the 75–85% range, and we suspect that these responses are constrained by modesty and by a wish not to appear boastful. Private, one-on-one conversations suggest that the true sense of confidence is often even higher.
A PC of 80% roughly corresponds to a correlation of .80. This level of predictive power is rarely achieved in the real world. In the field of personnel selection, a recent review found that the performance of human judges does not come close to this number. On average, they achieve a predictive correlation of .28 (PC = 59%).
Wherever there is prediction, there is ignorance, and more of it than you think.
Tetlock’s findings suggest that detailed long-term predictions about specific events are simply impossible. The world is a messy place, where minor events can have large consequences.
Models are consistently better than people, but not much better. There is essentially no evidence of situations in which people do very poorly and models do very well with the same information.
The order in which data about a claim becomes available varies haphazardly from one adjuster to the next and from one case to the next, causing random variation in initial impressions. Excessive coherence means that these random variations will produce random distortions in the final judgments. The effect will be system noise.
There is a genuine limit on people’s ability to assign distinct labels to stimuli on a dimension, and that limit is around seven labels.
There is a way to overcome the limited resolution of adjective scales: instead of using labels, use comparisons. Our ability to compare cases is much better than our ability to place them on a scale.
Behaviors are a function of personalities and of situations.
The average of errors (the bias) and the variability of errors (the noise) play equivalent roles in the error equation.
Good judgments depend on what you know, how well you think, and how you think. Good judges tend to be experienced and smart, but they also tend to be actively open-minded and willing to learn from new information.
Many professors, scholars, and management consultants are respect-experts. Their credibility depends on the respect of their students, peers, or clients. In all these fields, and many more, the judgments of one professional can be compared only with those of her peers.
In the absence of true values to determine who is right or wrong, we often value the opinion of respect-experts even when they disagree with one another.
You can be a young prodigy if your specialty is chess, concert piano, or throwing the javelin, because results validate your level of performance. But underwriters, fingerprint examiners, or judges usually need some years of experience for credibility. There are no young prodigies in underwriting.
One example is the Adult Decision Making Competence scale, which measures how prone people are to make typical errors in judgment like overconfidence or inconsistency in risk perceptions.
Another is the Halpern Critical Thinking Assessment, which focuses on critical thinking skills, including both a disposition toward rational thinking and a set of learnable skills.
The personality of people with excellent judgment may not fit the generally accepted stereotype of a decisive leader. People often tend to trust and like leaders who are firm and clear and who seem to know, immediately and deep in their bones, what is right. Such leaders inspire confidence. But the evidence suggests that if the goal is to reduce error, it is better for leaders (and others) to remain open to counterarguments and to know that they might be wrong. If they end up being decisive, it is at the end of a process, not at the start.
Ex post, or corrective, debiasing is often carried out intuitively. Suppose that you are supervising a team in charge of a project and that the team estimates that it can complete its project in three months. You might want to add a buffer to the members’ judgment and plan for four months, or more, thus correcting a bias (the planning fallacy) you assume is present.
Ex ante or preventive debiasing interventions fall in turn into two broad categories:
Noise reduction decision hygiene: When you wash your hands, you may not know precisely which germ you are avoiding—you just know that handwashing is good prevention for a variety of germs (especially but not only during a pandemic). Similarly, following the principles of decision hygiene means that you adopt techniques that reduce noise without ever knowing which underlying errors you are helping to avoid.
Other nudges work on different aspects of choice architecture. They might make the right decision the easy decision—for example, by reducing administrative burdens for getting access to care for mental health problems. Or they might make certain characteristics of a product or an activity salient—for example, by making once-hidden fees explicit and clear. Grocery stores and websites can easily be designed to nudge people in a way that overcomes their biases. If healthy foods are put in prominent places, more people are likely to buy them.
Decision observers in these cases fall in three categories.
Just as in the group decisions, an initial error prompted by confirmation bias becomes the biasing information that influences a second expert, whose judgment biases a third one, and so on.
The new procedures deployed in forensic laboratories aim to protect the independence of the examiners’ judgments by giving the examiners only the information they need, when they need it.
Linear sequential unmasking: examiners should document their judgments at each step. This sequence of steps helps experts avoid the risk that they see only what they are looking for. And they should record their judgment on the evidence before they have access to contextual information that risks biasing them.
When a different examiner is called on to verify the identification made by the first person, the second person should not be aware of the first judgment.
The easiest way to aggregate several forecasts is to average them. Averaging is mathematically guaranteed to reduce noise: specifically, it divides it by the square root of the number of judgments averaged. This means that if you average one hundred judgments, you will reduce noise by 90%, and if you average four hundred judgments, you will reduce it by 95%—essentially eliminating it. This statistical law is the engine of the wisdom-of-crowds approach.
A select-crowd strategy, which selects the best judges according to the accuracy of their recent judgments and averages the judgments of a small number of judges (e.g., five), can be as effective as straight averaging.
Also called estimate-talk-estimate, it requires participants first to produce separate (and silent) estimates, then to explain and justify them, and finally to make a new estimate in response to the estimates and explanations of others. The consensus judgment is the average of the individual estimates obtained in that second round.
This approach mirrors what is expected of forecasters in business and government, who should also be updating their forecasts frequently on the basis of new information, despite the risk of being criticized for changing their minds. (A well-known response to this criticism, sometimes attributed to John Maynard Keynes, is, “When the facts change, I change my mind. What do you do?”)
Rather than form a holistic judgment about a big geopolitical question (whether a nation will leave the European Union, whether a war will break out in a particular place, whether a public official will be assassinated), they break it up into its component parts. They ask, “What would it take for the answer to be yes? What would it take for the answer to be no?” Instead of offering a gut feeling or some kind of global hunch, they ask and try to answer an assortment of subsidiary questions.
Superforecasters systematically look for base rates.
“People should take into consideration evidence that goes against their beliefs” and “It is more useful to pay attention to people who disagree with you than to pay attention to those who agree.”
If you are assembling a team of judges, you should of course pick the best judge first. But your next choice may be a moderately valid individual who brings some new skill to the table rather than a more valid judge who is highly similar to the first one. A team selected in this manner will be superior because the validity of pooled judgments increases faster when the judgments are uncorrelated with one another than when they are redundant.
Most ratings of performance have much less to do with the performance of the person being rated than we would wish. As one review summarizes it, “the relationship between job performance and ratings of job performance is likely to be weak or at best uncertain.”
Structuring is an attempt to limit the halo effect, which usually keeps the ratings of one individual on different dimensions within a small range. (Structuring, of course, works only if the ranking is done on each dimension separately, as in this example: ranking employees on an ill-defined, aggregate judgment of “work quality” would not reduce the halo effect.)
If Lynn and Mary are evaluating the same group of twenty employees, and Lynn is more lenient than Mary, their average ratings will be different, but their average rankings will not. A lenient ranker and a tough ranker use the same ranks.
Frame-of-reference training, has been shown to help ensure consistency between raters. In this step, raters are trained to recognize different dimensions of performance. They practice rating performance using videotaped vignettes and then learn how their ratings compare with “true” ratings provided by experts. The performance vignettes act as reference cases; each vignette defines an anchor point on the performance scale, which becomes a case scale,
With a case scale, each rating of a new individual is a comparison with the anchor cases. It becomes a relative judgment. Because comparative judgments are less susceptible to noise than ratings are, case scales are more reliable than scales that use numbers, adjectives, or behavioral descriptions.
Given the preceding levels of correlation, if all you know about two candidates is that one appeared better than the other in the interview, the chances that this candidate will indeed perform better are about 56 to 61%. Somewhat better than flipping a coin, for sure, but hardly a fail-safe way to make important decisions.
Why do first impressions end up driving the outcome of a much longer interview? One reason is that in a traditional interview, interviewers are at liberty to steer the interview in the direction they see fit. They are likely to ask questions that confirm an initial impression. If a candidate seems shy and reserved, for instance, the interviewer may want to ask tough questions about the candidate’s past experiences of working in teams but perhaps will neglect to ask the same questions of someone who seems cheerful and gregarious.
Interviewers with positive first impressions, for instance, ask fewer questions and tend to “sell” the company to the candidate.
However much we would like to believe that our judgment about a candidate is based on facts, our interpretation of facts is colored by prior attitudes.
Google stringently enforces a rule that not all companies observe: the company makes sure that the interviewers rate the candidate separately, before they communicate with one another. Once more: aggregation works—but only if the judgments are independent.
To test job-related knowledge, it relies in part on work sample tests, such as asking a candidate for a programming job to write some code. Research has shown that work sample tests are among the best predictors of on-the-job performance.
Google also uses “backdoor references,” supplied not by someone the candidate has nominated but by Google employees with whom the candidate has crossed paths.
Do not exclude intuition, but delay it.
Google allows judgment and intuition in its decision-making process only after all the evidence has been collected and analyzed. Thus, the tendency of each interviewer (and hiring committee member) to form quick, intuitive impressions and rush to judgment is kept in check.
“We should decide in advance on a list of assessments of different aspects of the deal, just as an interviewer starts with a job description that serves as a checklist of traits or attributes a candidate must possess. We will make sure the board discusses these assessments separately, one by one, just as interviewers in structured interviews evaluate the candidate on the separate dimensions in sequence. Then, and only then, will we turn to a discussion of whether to accept or reject the deal. This procedure will be a much more effective way to take advantage of the collective wisdom of the board.
“Using a structured approach will force us to postpone the goal of reaching a decision until we have made all the assessments. We will take on the separate assessments as intermediate goals. This way, we will consider all the information available and make sure that our conclusion on one aspect of the deal does not change our reading on another, unrelated aspect.”
“The first thing we are going to do,” Joan explained, “is draw up a comprehensive list of independent assessments about the deal. These will be assessed by Jeff Schneider’s research team. Our task today is to construct the list of assessments. It should be comprehensive in the sense that any relevant fact you can think of should find its place and should influence at least one of the assessments. And what I mean by ‘independent’ is that a relevant fact should preferably influence only one of the assessments, to minimize redundancy.”
The deal team’s mission, as Joan saw it, was not to tell the board what it thought of the deal as a whole—at least, not yet. It was to provide an objective, independent evaluation on each of the mediating assessments.
The evaluations should be based on facts—nothing new about that—but they should also use an outside view whenever possible.
They would need to start by finding out the base rate. This task would, in turn, require them to define a relevant reference class, a group of deals considered comparable enough.
Jeff then explained how to evaluate the technological skills of the target’s product development department—another important assessment Joan had listed. “It is not enough to describe the company’s recent achievements in a fact-based way and to call them ‘good’ or ‘great.’ What I expect is something like, ‘This product development department is in the second quintile of its peer group, as measured by its recent track record of product launches.’ ” Overall, he explained, the goal was to make evaluations as comparative as possible, because relative judgments are better than absolute ones.
If there is information that seems inconsistent or even contradictory with the main rating, don’t sweep anything under the rug. Your job is not to sell your recommendation. It is to represent the truth. If it is complicated, so be it—it often is.”
Estimate-talk-estimate method, which combines the advantages of deliberation and those of averaging independent opinions.
Joan asked the board members to use a voting app on their phones to give their own rating on the assessment. The distribution of ratings was projected immediately on the screen, without identifying the raters. “This is not a vote,” Joan explained. “We are just taking the temperature of the room on each topic.” When the discussion of an assessment drew to a close, Joan asked the board members to vote again on a rating.
“This is not just about computing a simple combination of the assessment ratings,” she said. “We have delayed intuition, but now is the time to use it. What we need now is your judgment.”
Reducing noise can be expensive; it might not be worth the trouble. The steps that are necessary to reduce noise might be highly burdensome. In some cases, they might not even be feasible.
Some strategies introduced to reduce noise might introduce errors of their own.
If all doctors at a hospital prescribed aspirin for every illness, they would not be noisy, but they would make plenty of mistakes.
If we want people to feel that they have been treated with respect and dignity, we might have to tolerate some noise.
If we eliminate noise, we might reduce our ability to respond when moral and political commitments move in new and unexpected directions. A noise-free system might freeze existing values.
Some strategies designed to reduce noise might encourage opportunistic behavior, allowing people to game the system or evade prohibitions. A little noise, or perhaps a lot of it, might be necessary to prevent wrongdoing.
If people know that they could be subject to either a small penalty or a large one, they might steer clear of wrongdoing, at least if they are risk-averse. A system might tolerate noise as a way of producing extra deterrence.
People do not want to be treated as if they are mere things, or cogs in some kind of machine. Some noise-reduction strategies might squelch people’s creativity and prove demoralizing.
A noise-free scoring system that fails to take significant variables into account might be worse than reliance on (noisy) individual judgments.
Although a predictive algorithm in an uncertain world is unlikely to be perfect, it can be far less imperfect than noisy and often-biased human judgment. This superiority holds in terms of both validity (good algorithms almost always predict better) and discrimination (good algorithms can be less biased than human judges).
Some people might insist that an advantage of a noisy system is that it will allow people to accommodate new and emerging values. As values change, and if judges are allowed to exercise discretion, they might begin to give, for example, lower sentences to those convicted of drug offenses or higher sentences to those convicted of rape.
Unfairness might be tolerated if it allows room for novel or emerging social values.
We need to monitor our rules to make sure they are operating as intended. If they are not, the existence of noise might be a clue, and the rules should be revised.
For repeated decisions, there are real advantages to moving in the direction of mechanical rules rather than ad hoc judgments. The burdens of exercising discretion turn out to be great, and the costs of noise, or the unfairness it creates, might well be intolerable.